Article Details : |
| | Article Name : | | Bootstrap and multiple imputation under missing
data in AR(1) models | Author Name : | | ELJONA MILO, LORENA MARGO
| Publisher : | | Bridge Center | Article URL : | 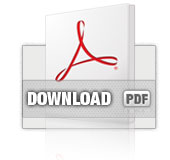 | | Abstract : | | Missing data is a phenomenon detected in many scientific
investigations due to the bias often caused and inefficient analysis of
the data. Determining the appropriate analytic approach in the
presence of incomplete observations is a major question for data
analysts. Recently many scientists have developed several statistical
methods to address missingness.
Since the introduction by Efron (1979), bootstrap has resulted
to be an important method for estimating the distribution of an
estimator by applying the resampling of the data. This bootstrap
method resulted efficient in the case of independent and identically
distributed observations, but in the case of dependent data like time
series, classic bootstrap gives incorrect answers. For this reason, to
adapt the bootstrap in the case of time series Kunsch (1989) presented
a bootstrap method with blocks compounded by a fixed number of
observations. Block bootstrap methods developed by researchers
resulted suitable in the case of time series and give good results under
specific assumptions.
In this paper we will realize simulations with intention to
compare the results obtained using block bootstrap combined with
missing data mechanisms. We are interested in estimating the
parameter in the AR(1) time series model using block bootstrap
procedure after filling in the missing values using multiple
imputation. We compare the results using several block length also
different missing data mechanisms and packages for completing the
missing values. | Keywords : | | Bootstrap, time series, missing data, imputation,
autoregressive |
|
|
|
|
|
|
Announcements
|
- Volume 9, issue 5, August issue - publishing in progress
- Volume 9, Issue 2/ May 2021 publishing IN PROGRESS
- Call for Papers: open. Articles will be uploaded constantly, once the evaluation process is complete.
- The Certificates of Publication can be downloaded from RECEIVED ARTICLES section.
- COVER - VOLUME 2, ISSUE 12 / MARCH 2015
- COVER - VOLUME 3, ISSUE 2 / MAY 2015
- COVER - VOLUME 3, ISSUE 3 / JUNE 2015
- COVER - VOLUME 3, ISSUE 4 / JULY 2015
- COVER - VOLUME 3, ISSUE 8/ NOVEMBER 2015
- COVER - VOLUME 4, ISSUE 4 / JULY 2016
- COVER - VOLUME 4, ISSUE 5 / AUGUST 2016
- COVER - VOLUME 7, ISSUE 11/ FEBRUARY 2020
- Volume 9, issue 3, June 2021 publishing in progress
|
|
New Launched Project |
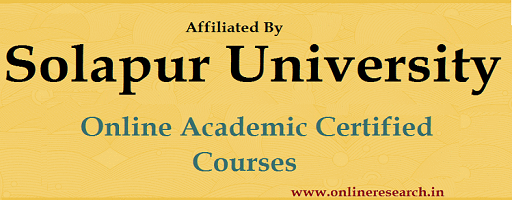 |
|
Recommend & Share
|
|