Article Details : |
| | Article Name : | | A Comparative Study on Machine Learning Tools
Using WEKA and Rapid Miner with Classifier
Algorithms C4.5 and Decision Stump for Network
Intrusion Detection | Author Name : | | WATHQ AHMED ALI SAEED KAWELAH, AHMED SALAH ELDIN ABDALA | Publisher : | | Bridge Center | Article URL : | 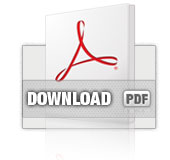 | | Abstract : | | Intrusion detection system dealing with the huge amount of
data that include repeated irrelevant cause slow process of testing,
training and higher learning resource consumption as well as the
vulnerability of the detection rate. Data mining techniques are being
applied in the construction of intrusion detection systems to protect
computing resources against unauthorized access. In this paper, we
have done a comparative study on machine learning tools using WEKA
and Rapid Miner with two classifier algorithms C4.5 and Decision
Stump for Network Intrusion Detection to measure the accuracy,
sensitivity and precision. The results of the experiments using the
KDD’ 99 attack dataset and select seven features, The results show the
best tools Rapid Miner for the accuracy and precision, while the best
algorithms is C4.5.
| Keywords : | | Data Mining Tools; WEKA; Rapid Miner; C4.5 and
Decision Stump |
|
|
|
|
|
|
Announcements
|
- Volume 9, issue 5, August issue - publishing in progress
- Volume 9, Issue 2/ May 2021 publishing IN PROGRESS
- Call for Papers: open. Articles will be uploaded constantly, once the evaluation process is complete.
- The Certificates of Publication can be downloaded from RECEIVED ARTICLES section.
- COVER - VOLUME 2, ISSUE 12 / MARCH 2015
- COVER - VOLUME 3, ISSUE 2 / MAY 2015
- COVER - VOLUME 3, ISSUE 3 / JUNE 2015
- COVER - VOLUME 3, ISSUE 4 / JULY 2015
- COVER - VOLUME 3, ISSUE 8/ NOVEMBER 2015
- COVER - VOLUME 4, ISSUE 4 / JULY 2016
- COVER - VOLUME 4, ISSUE 5 / AUGUST 2016
- COVER - VOLUME 7, ISSUE 11/ FEBRUARY 2020
- Volume 9, issue 3, June 2021 publishing in progress
|
|
New Launched Project |
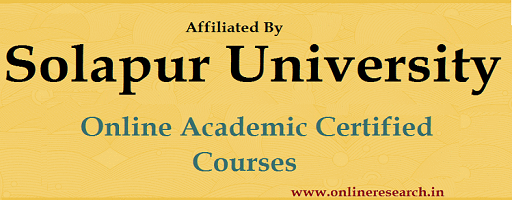 |
|
Recommend & Share
|
|