Article Details : |
| | Article Name : | | Classification of Anomalous Gait Using Machine Learning Techniques and Embedded Sensors | Author Name : | | Tiago R. de Sá, Carlos M. S. Figueiredo | Publisher : | | Bridge Center | Article URL : | 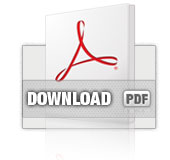 | | Abstract : | | Human gait can be a predictive factor for detecting pathologies that affect human locomotion according to studies. In addition, it is known that a high investment is demanded in order to raise a traditional clinical infrastructure able to provide human gait examinations, making them unaffordable for economically vulnerable patients. In face of this scenario, we proposed an accessible and modern solution composed of a wearable device in order to collect 3D-accelerometer and 3D-gyroscope data. In addition, we also presented machine learning techniques to classify between distinct categories of induced gait disorders. In order to develop this proposed research, we created a dataset with the target label being four distinct and balanced categories of anomalous gait. By using Principal Component Analysis algorithm with Support Vector Machines classifier, we achieved the best performance (94 % accuracy) in this dataset when comparing it with other traditional machine learning techniques. Further, we developed a Feedforward Neural Network model based that yielded even better results (96 % accuracy). Finally, we also presented computational performance comparison between the models implemented. | Keywords : | | Human Gait, Machine Learning, Internet of Things, Wearables, Healthcare. |
|
|
|
|
|
|
Announcements
|
- Volume 9, issue 5, August issue - publishing in progress
- Volume 9, Issue 2/ May 2021 publishing IN PROGRESS
- Call for Papers: open. Articles will be uploaded constantly, once the evaluation process is complete.
- The Certificates of Publication can be downloaded from RECEIVED ARTICLES section.
- COVER - VOLUME 2, ISSUE 12 / MARCH 2015
- COVER - VOLUME 3, ISSUE 2 / MAY 2015
- COVER - VOLUME 3, ISSUE 3 / JUNE 2015
- COVER - VOLUME 3, ISSUE 4 / JULY 2015
- COVER - VOLUME 3, ISSUE 8/ NOVEMBER 2015
- COVER - VOLUME 4, ISSUE 4 / JULY 2016
- COVER - VOLUME 4, ISSUE 5 / AUGUST 2016
- COVER - VOLUME 7, ISSUE 11/ FEBRUARY 2020
- Volume 9, issue 3, June 2021 publishing in progress
|
|
New Launched Project |
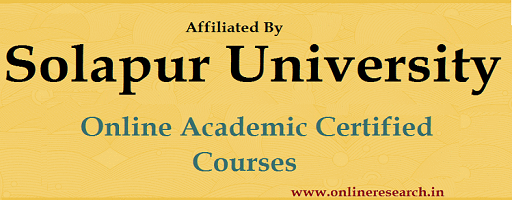 |
|
Recommend & Share
|
|